Business data comprises facts and figures that a company processes that is used to measure a wide range of business activities for both internal and external purposes. Business data needs to be interpreted and presented in a more meaningful context to allow businesses to make better decisions. With the help of document conversion services, paper-based data can be transformed to digital format for long term use.
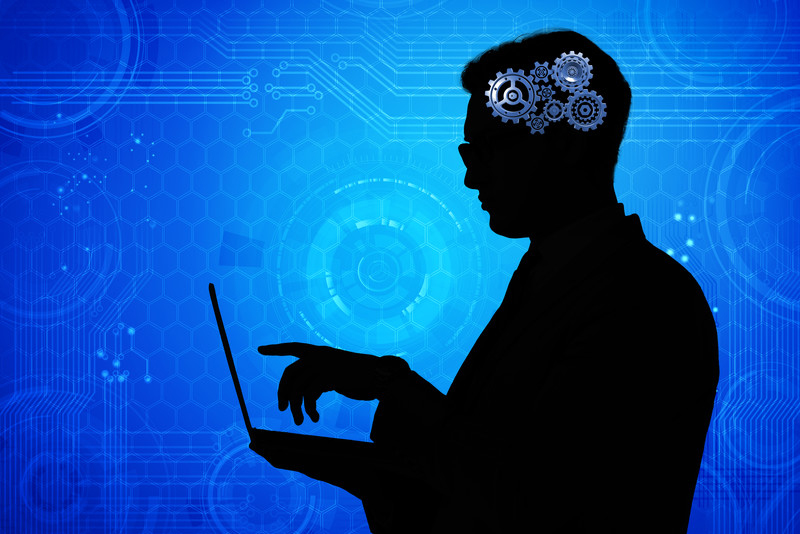
Implementing a digital business strategy is crucial and complex. Innovations in digitization and robotization are quickly gaining foothold in all industries, including healthcare. Companies can improve productivity if they skilfully manage the automation transformation. Organizations are increasingly using artificial intelligence to identify anomalies, make suggestions, and solve problems. As the annual 2019 HIMSS Conference and Exhibition highlighted, artificial intelligence has become a necessity now rather than a mere novelty.
In 2018, the audience were rather sceptical and EHR vendors as well as big data experts found it highly challenging to convince them about the AI advantage. Many users had experienced unintentional negative impacts of AI solutions and were cynical. This year, potential investors demand proof that AI can ensure prescriptive insights and help them in their decision making. They want to be sure that those insights can help them anticipate and prevent future adverse events. This is a positive development.
The major challenge healthcare entities have to overcome before implementing AI is data aggregation. They need to access huge volumes of files, and extract clean, complete, and timely data that is required to train, authenticate and deploy AI for practical use. For effective AI implementation, healthcare organizations should ensure data integration.
Data Integration
According to Mark Morsch, Vice President of Technology at Optum healthcare, organizations have entered a crucial year for AI and advanced analytics. The year 2019 is the year for adoption and maturity of AI. More and more organizations are moving from diagnostics and descriptive level to more predictive and prescriptive level and people are appreciating the challenges and opportunities of putting AI into practice. The shift to AI is optimizing clinical workflows and improving operational efficiencies with machine learning as part of the toolset.
Data science is not a new concept but the problem is the readiness of the healthcare industry to implement the technology. AI resolves unstructured healthcare data and merges into structured data to extract predictive instead of retrospective results. However, data silos are a major issue. Similarly, breaking down the barriers to data access and adhering to privacy and security principles is a huge challenge.
Another concern is interoperability. To achieve smooth interoperability, purposeful information blocking must be prevented. There should be greater access to the required data for purposes such as population health management, patient engagement, and quality enhancement. According to David Dimond, Chief Technology Officer and Distinguished Engineer at Dell EMC’s Global Healthcare Business, analyzing a particular data set with AI can become useful only if you can build a health record around it and develop a longitudinal view of an individual’s health. Healthcare organizations have too much data in many different places and for effective implementation of AI, data integration is inevitable.
Accumulating Fragmented Data
According to a 2017 survey by Accenture, more than 80 percent of healthcare executives have started working on creating centralized data platforms to strengthen their artificial intelligence plans. 82 percent believed that a good leader in the modern health IT landscape can architect seamless, interoperable environments for conducting large-scale analytics. However, challenges such as existing legacy systems, disappointing EHR implementation decisions, shortage of money and time to tackle data integrity from the bottom up, and the large number of potential data sources are leading to data silos. This makes data access difficult. Most of the medical data is unstructured and retrieving it and organizing it is burdensome.
Machine learning could help with structuring the incoherent data.
- As a first step, an OCR algorithm is run to turn an image into PDF and then NLP is used to extract meaningful elements from that or identify patterns you might be looking for.
- Since this first step itself is challenging, businesses can consider joining hands with a strong technology partner that can help them leverage AI for the best measurable results.
- NLP can be coupled with deep learning, for example to streamline the process of medical chart review in a healthcare setting.
- Deep learning can review each case and identify those cases that should be reviewed by a human expert as well.
- With the right data, the technology can transform the process of reviewing cases for medical coding and billing as well.
- Major steps in AI implementation include understanding the present workflow, identifying the opportunities to improve workflow, and then applying machine learning to those concerns.
Healthcare organizations must develop an adaptable and practical business strategy and combine it with aptly aggregated data and AI to maximize the advantages. Application areas include patient scheduling, staffing, and reallocating resources. AI solutions can identify all factors that may affect patient care so that the necessary steps can be taken in a timely manner to prevent any adverse event.
Setting Up the Technical Foundation for Data Integration
EHR vendors need an infrastructure strategy to get hold of all data and prioritize aggregation while protecting the privacy and security of data. In 2019, HIMSS conference attendees were asked to focus on cloud storage and computing to enable the next generation of data analytics. Cloud storage system providers are marketing their AI capabilities to the healthcare industry. Prefabricated machine learning models and toolkits are being provided by Google and Amazon Web Service. Once the healthcare organization has become successful in data aggregation, the cloud system will become the default for AI-enabled organizations in the future. Healthcare organizations now rely on cloud storage providers rather than investing billions into security protocols for safeguarding their data.
With cloud storage, individual organizations can aggregate their data in a single location or link together data existing in multiple locations. It also creates the opportunity for multiple organizations to collaborate on developing and validating machine learning models.
There are many opportunities for healthcare organizations for data aggregation and creating the right environment for machine learning. Cloud is an important tool and it is something that all healthcare organizations should consider as they grow. Implementing the technical foundation for Artificial Intelligence is important if healthcare organizations are to continue their journey towards better data analytics and use.
Digitization, the first step towards data aggregation is most important. Gathering all medical data and transforming it into digital format with the help of data conversion services enables quick access to data, efficient data analytics, and deriving valuable business insights.