Like any other industry, the banking and other financial industry sector is automating their services by implementing online banking system, mobile payments etc. However, there are still many financial document processing needs that are met with the support of data entry services. While bulk document scanning continues to be essential across the financial industry, automation being implemented in the industry helps to generate an improvement of more than 50 percent in productivity and customer service.
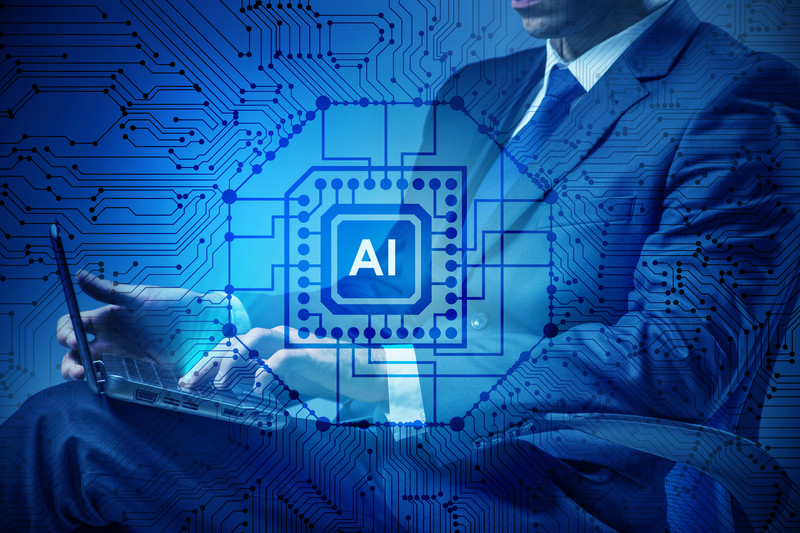
Financial Institutions are in the initial stage of adopting Artificial Intelligence (AI). Estimates are that 75 percent of insurance companies feel that AI will either alter or transform the industry. AI will help financial institutions to redefine the way they gain information from various sources, and also assures better data analysis, insights and customer satisfaction. Goldman Sachs, one of the leading global financial institutions, has embraced AI with good results. They implemented automation in various areas like currencies and futures using complex trading algorithms, some with machine learning. According to an MIT review, the number of cash equities trading desk at Goldman Sach’s New York headquarters employees went from 600 in 2000 to just 2 equity traders and automated trading programs assisted by 200 computers engineers doing the rest of the work. Other banking institutions that have implemented AI or ML (Machine Learning) are ICICI, HDFC, BofA, Schwab and JP Morgan.
Insurance companies like Aliianz, MetLife, QBE Insurance Group, XL Catlin and Aetna are also adopting different applications of AI. MetLife reports that Shift Technology, an AI start-up based in France, helped a European coalition of insurers to analyze 13 million claims. This technology identified 3,000 new cases of potential fraud, which included crime schemes that nearly impacted all the coalition members. Although financial industries are relying on AI or ML, the efficiency of all new technology depends on clean data.
The Need for Clean Data for Successful Adoption of AI
The success of Machine Learning and Artificial Intelligence depends on accurate data. Clean and reliable data is necessary to derive valuable insights that enables effective decision making. Even though smart technologies are beneficial for various elements of the value chain and operations, financial institutions are yet to exploit the potential of AI due to the complexities involved in organizing data. This highlights the importance of data cleansing outsourcing services.
Jon Theuerkauf, former managing director and group head of performance excellence at BNY says that the key to AI is structured data. Today, 80 percent of modern data is unstructured which poses a security and it restricts the company from adopting smart technologies. The main challenge the financial industry faces is to extract valuable insights from unstructured data and to process the huge volumes of data. Another problem is that unstructured data will increase the chances for cyber attack. According to IBM, businesses are struggling to understand unstructured data, how it can be used, who can access the data etc. The most difficult step is to apply a standard measure to protect the data.
Structured and organized data is important for dynamic and accurate adjustment of financial products and services to determine the changing consumer preferences and market conditions. Structured data is the competitive advantage for 40 percent of the G-SIFIs(global systemically important financial institutions) in the US. Financial institution scan rely on data cleansing services to transform unstructured data into structured data, which would make data analytics easier and more efficient.
A Human Touch Brings More Accuracy
No matter how advanced the industry’s data driven methods are, it still requires a human touch to ensure that the algorithms work efficiently. This shows that even though use of AI intelligence easily automates data, institutions may still need the assistance of data entry services. They help financial institutions to transform unstructured data into structured data sets that enable smart technologies to analyze and process data and also minimize the chances risk and errors.